Navigating the Future of Healthcare: The Role of Machine Learning in Enhancing Patient Outcomes
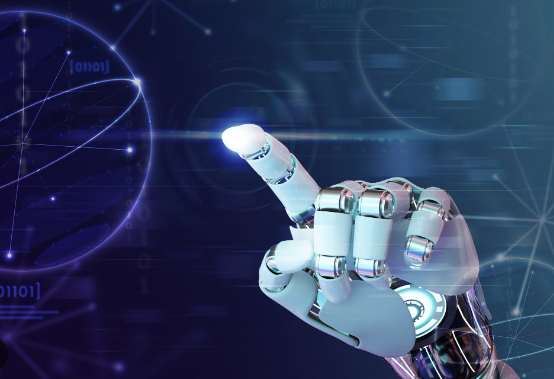
The healthcare sector has always stood at the forefront of technological advancement, embracing innovations that can elevate the quality of patient care. Among these technological shifts, the emergence of machine learning in healthcare stands out for its profound potential to transform patient outcomes. As algorithms become more predictive and responsive, the face of medicine is changing, steering toward a future where data-driven decisions are commonplace and individual patient care is the norm, not the exception.
Understanding Machine Learning in Healthcare
Machine learning, a branch of artificial intelligence that trains computers to interpret data, find patterns, and make decisions with minimal human intervention, is carving its niche within the healthcare industry. This cutting-edge technology plays a crucial role in processing the enormous volume of patient data and turning it into insights that may be used to guide early intervention and effective treatment choices. It is becoming increasingly clear that machine learning in healthcare will play a significant role in the future as our understanding of it and its capabilities expands.
Technology Meets Medicine: The Benefits of Integrating Machine Learning
In the complex healthcare environment, machine learning technologies shine as they contribute to more nuanced and robust care delivery processes. These emerging systems help manage the deluge of patient data, crafting individualized treatment plans and ensuring that healthcare professionals are equipped to make more informed decisions. By automating routine tasks and deciphering complex data sets, machine learning frees clinicians to focus on the nuances of patient care, thus enhancing the overall quality of service. Moreover, the technology’s ability to uncover insights across large populations aids in the early detection of epidemics and tailoring public health strategies.
Machine Learning’s Impact on Diagnosis and Treatment Plans
The ability of machine learning models to sift through immense datasets and identify patterns undetectable to the human eye has significant ramifications for diagnostic accuracy. Its augmented analytical ability leads to the potential for earlier recognition of conditions, which is paramount in diseases where early detection is linked to better outcomes. In the realm of treatment, personalized medicine is becoming a reality as algorithms factor in individual genetic makeup, lifestyle, and even social determinants to suggest optimal treatment modalities. The result is a healthcare landscape where treatments are tailored, outcomes are improved, and patients are empowered.
Patient-Centered Care: How Machine Learning Contributes
Machine learning pushes the healthcare industry towards a patient-centered model, fostering a collaborative environment where patients are key decision-makers in their treatment plans. Patient engagement has never been higher, thanks to tools that provide real-time health monitoring and feedback. With the steady advent of wearable technology and health apps, machine learning creates a continuous loop of patient data that significantly augments preventive medicine and chronic disease management. Patients can now better manage and understand their health, supported by intelligent systems that can alert, advise, and assist in everyday health-related decisions.
From Data to Decisions: Machine Learning’s Analytical Power
Healthcare data is complex, diverse, and voluminous. Through their superb pattern recognition capabilities, machine learning algorithms are increasingly successful in extrapolating valuable insights from this data deluge. This analytical power assumes critical importance in clinical decision-making processes, enhancing the reliability and timeliness of medical interventions. Moreover, by predicting patient admissions and potential health risks, machine learning stands poised to effect significant cost reductions and resource optimizations within healthcare systems around the globe.
Overcoming Challenges: Privacy, Ethics, and Data Security
The integration of machine learning into healthcare has its challenges. Privacy concerns, data security, and ethical dilemmas present significant hurdles that must be navigated with care. As machines access sensitive information, ensuring adequate safeguards to protect patient confidentiality becomes imperative. Furthermore, ethical considerations around AI decision-making, bias in algorithmic processes, and the autonomy of AI in healthcare settings necessitate clear guidelines and regulations. Addressing these concerns is critical in maintaining the integrity and trust in technology-assisted healthcare services. The article on ethical challenges posed by AI in healthcare underscores the importance of aligning machine learning applications with the ethical standards expected in medical practice.
Future Prospects: Ongoing Research and Development
As machine learning evolves, so does the medical research and development landscape. Emerging studies and initiatives are shedding light on new applications and efficiencies this technology can bring to healthcareāthese range from enhancing drug discovery processes to providing real-time support in surgical settings. Furthermore, the cross-pollination of ideas and expertise between technologists and medical professionals fosters a fertile ground for future breakthroughs. This collaboration propels the technology forward and ensures its relevancy and application in real-world medical scenarios.